Brian R.Y. Huang
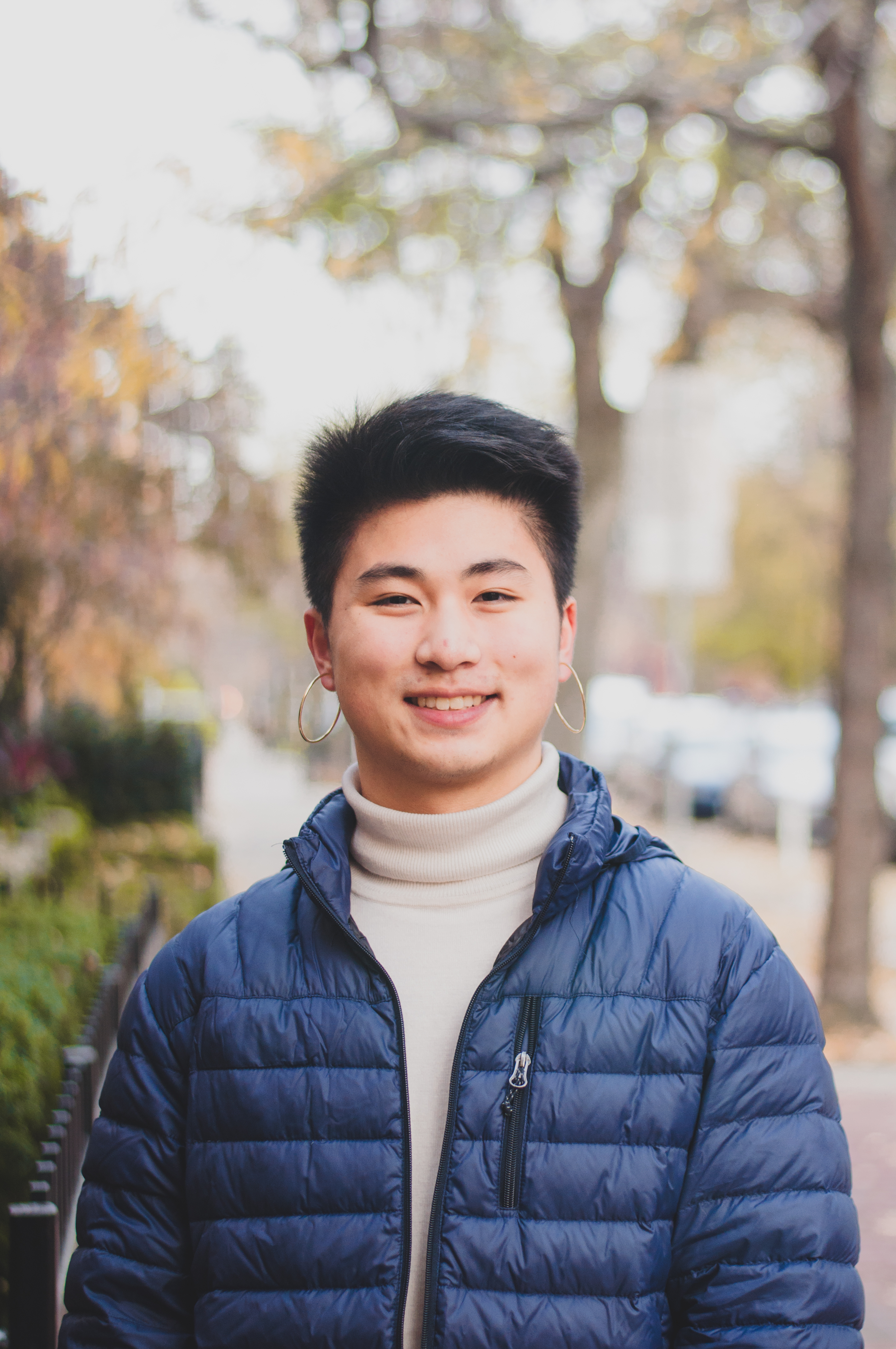
I’m a recent graduate from MIT with Bachelor’s degrees in Math & CS as well as a Master’s in CS. I’m broadly interested in methods and evaluations that may engender more helpful, equitable, and harm-reducing outcomes for all stakeholders of frontier AI systems.
At MIT, I was fortunate to conduct research on adversarial robustness of deep learning models, advised by Hadi Salman and Aleksander Mądry. I was also a teaching assistant for MIT’s flagship graduate-level machine learning class (6.867, now 6.7900) and for the statistical data analysis class (6.3720/3722). Before undergrad, I researched black hole formation in general relativity with Marcus Khuri at Stony Brook University. I’ve also worked on causal mediation analysis for mechanistic interpretability as a research intern at Redwood Research. I’m currently working on interesting perception problems as a research engineer at Matic. Post-graduation, I’ve continued my involvement in various research collaborations, studying factuality, adversarial inputs, and other phenomena in language models.
I’m always open to nuanced discussions about safe, equitable, and rigorously guardrailed deployment of AI systems, as well as the challenges in tech policy and “science of language models” that we must address along the way. I’d also be excited to collaborate on research along the lines of, but not limited to, my research directions here. if our research interests align, feel free to reach out at branhung (at) alum (dot) mit (dot) edu!
selected works
- Does It Know?: Probing and Benchmarking Uncertainty in Language Model Latent BeliefsATTRIB Workshop @ NeurIPS, 2023
- Adversarial Learned Soups: Neural Network Averaging for Joint Clean and Robust PerformanceMaster’s Thesis, 2023
We introduce weight-space interpolation methods to the adversarial robustness regime, devising a wrapper architecture to optimize the interpolation coefficients of a "model soup" via adversarial training. Varying the intensity of adversarial training (perturbation distance, TRADES weightings, etc.) leads to a smooth tradeoff between the resulting clean and robust accuracy of the interpolated model.