Brian R.Y. Huang
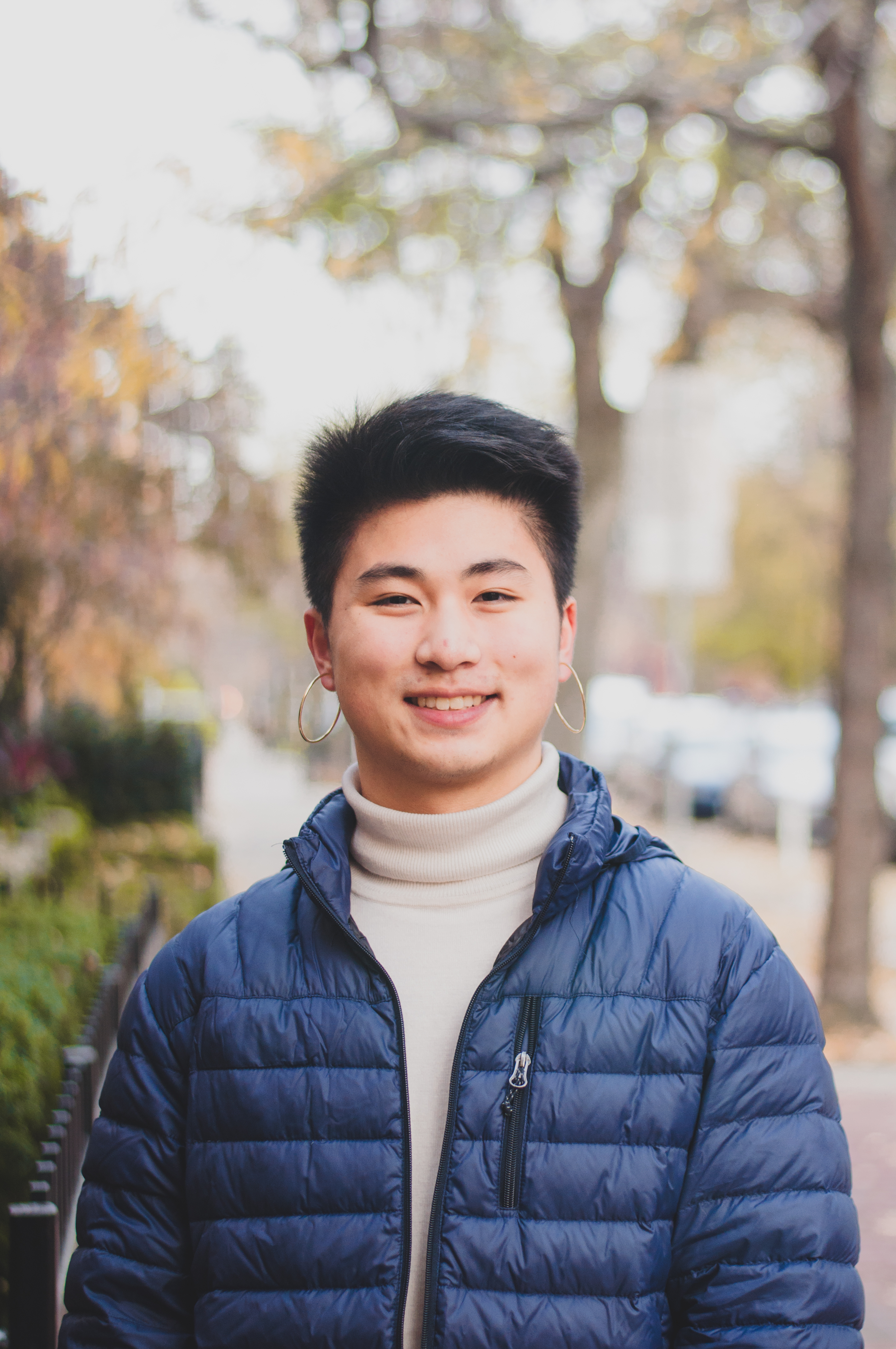
I’m a recent graduate from MIT with Bachelor’s degrees in Math & CS as well as a Master’s in CS. I’m broadly interested in understanding failure modes and weaknesses in frontier AI systems in order to robustify real-world deployment of AI systems.
At MIT, I was fortunate to conduct research on adversarial robustness of deep learning models, advised by Hadi Salman and Aleksander Mądry. I was also a teaching assistant for MIT’s flagship graduate-level machine learning class (6.867, now 6.7900) and for the statistical data analysis class (6.3720/3722). In past internships/research experiences, I’ve worked on causal intervention methods for mech interp at Redwood Research; researched ~quantitatively~ at JPMorgan Chase and WorldQuant; and investigated differential-geometric properties of black hole formation in general relativity with Marcus Khuri at Stony Brook University. Most recently, I was an AI resident at Haize Labs doing research and engineering for red-teaming, automated evals, and adversarial robustness.
selected works
- Endless Jailbreaks with Bijection LearningICLR, 2025
We devise a "bijection attack," an encoding scheme taught to a language model in-context which bypasses model alignment and comprises a highly effective jailbreak. We differentially modulate the complexity of our bijection scheme across different models and derive a quadratic scaling law, finding that, curiously, our bijection attack is stronger on higher-capability models. - Adversarial Learned Soups: Neural Network Averaging for Joint Clean and Robust PerformanceMaster’s Thesis, 2023
We introduce weight-space interpolation methods to the adversarial robustness regime, devising a wrapper architecture to optimize the interpolation coefficients of a "model soup" via adversarial training. Varying the intensity of adversarial training (perturbation distance, TRADES weightings, etc.) leads to a smooth tradeoff between the resulting clean and robust accuracy of the interpolated model. - Does It Know?: Probing and Benchmarking Uncertainty in Language Model Latent BeliefsATTRIB Workshop @ NeurIPS, 2023
- On Sufficient Conditions for Trapped Surfaces in Spherically Symmetric Spacetimespresented at Siemens Competition, 2017